Understanding Decision Making Models Explained
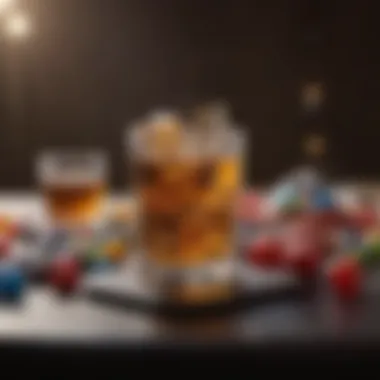
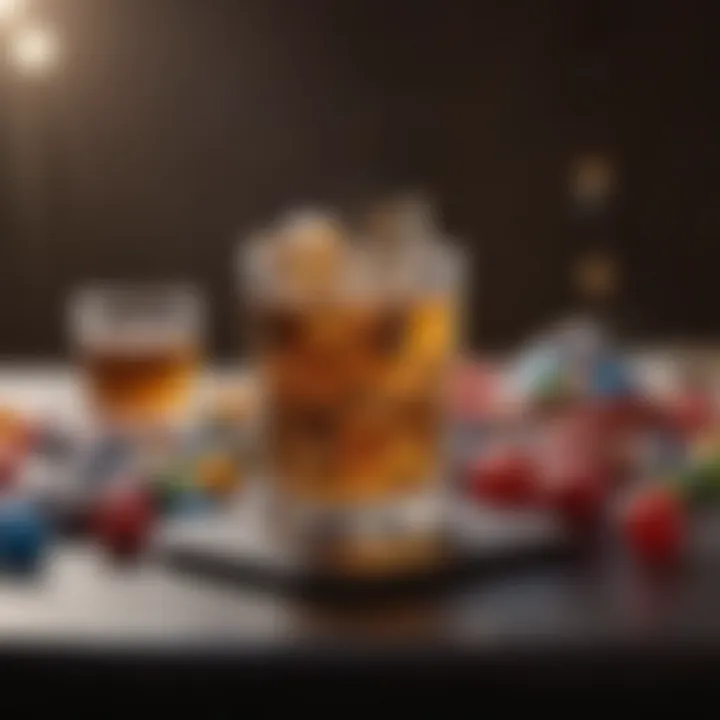
Intro
Decision-making is a fundamental aspect of both personal and professional life. As we traverse through myriad choices daily, understanding the mechanisms that underpin these decisions can significantly influence outcomes. This guide explores various decision-making models that can streamline the process, making it more systematic and effective.
The models we analyze not only highlight the different approaches to decision-making but also illustrate their real-world applications. Our journey will also touch upon how these models are integrated with artificial intelligence and behavioral science. Understanding these elements can yield insights that enhance both individual and organizational effectiveness.
Understanding Resilience, Well-Being, and Adaptability
Resilience, well-being, and adaptability are crucial concepts within the realm of personal and organizational development. At their core, resilience is the ability to bounce back from setbacks, well-being refers to an individual's overall mental health and life satisfaction, and adaptability is the capacity to adjust to new conditions.
Importance of Resilience, Well-Being, and Adaptability
These factors are interconnected and play a significant role in enhancing an individual's ability to make informed decisions. In organizations, fostering resilience and well-being among employees leads to improved morale and productivity. A workplace that values adaptability is better equipped to navigate change and uncertainty, ultimately driving success.
Strategies for Cultivating Resilience and Well-Being
- Self-Reflection: Encourage individuals to analyze their responses to challenges.
- Support Networks: Promote the formation of strong support systems among colleagues and friends.
- Mindfulness Training: Implement practices that enhance present-moment awareness, reducing stress.
- Goal Setting: Teach individuals to set achievable goals, fostering a sense of accomplishment.
"Strength does not come from physical capacity. It comes from an indomitable will."
Integration of AI Technology in Coaching
Artificial intelligence has begun to reshape various industries, including coaching. AI technology offers innovative solutions that enhance the way individuals and organizations approach development.
Benefits of AI-Driven Coaching Solutions
- Personalization: Tailors coaching experiences based on individual needs and preferences.
- Efficiency: Increases the speed and effectiveness of decision-making processes.
- Data-Driven Insights: Provides actionable insights derived from analytics, helping both coaches and clients.
Case Studies Highlighting Successful Implementation
A few organizations have adopted AI-driven coaching platforms, yielding notable results. For instance, platforms like BetterUp have successfully integrated AI to provide personalized coaching experiences that adapt to clients' evolving needs.
Leveraging Behavioral Science for Personal Growth
Behavioral science offers valuable insights into the underlying factors that drive our decisions. Understanding these principles can aid in formulating strategies for personal growth.
Key Principles of Behavioral Science
- Cognitive Bias: Recognizing biases helps in making more objective decisions.
- Nudge Theory: Small design changes can influence behavior positively.
Practical Applications of Behavioral Science
- Habit Formation: Encourage daily routines that support desired behaviors.
- Environmental Adjustments: Modify surroundings to foster productivity and well-being.
Techniques Based on Behavioral Science
- Utilizing visualization techniques to enhance performance.
- Engaging in regular self-assessment to promote continual growth.
Interactive Tools for Self-Improvement
Various interactive tools are available for individuals seeking personal growth. These platforms often provide engaging methods to enhance resilience, adaptability, and overall well-being.
Overview of Interactive Tools
Tools such as Headspace for mindfulness and Duolingo for language learning present practical applications for personal development and improvement.
Effective Utilization of Tools
To maximize the benefits, individuals should establish a routine that integrates these tools into their daily lives. Consistency is key to achieving significant improvements.
User Testimonials
Many users report increased motivation and clarity in their personal and professional lives after using interactive tools. The impact varies by individual, but the common denominator is a more empowered state of being.
Defining Decision Making Models
Decision making is a fundamental aspect of both personal and organizational contexts. It influences the paths individuals and groups take to achieve goals. Defining decision making models serves as the foundation for understanding how decisions are made, providing clarity in complex situations and enabling more efficient choices.
These models summarize various approaches to making decisions, translating intricate processes into frameworks that can guide people toward better outcomes. By delineating stages, inputs, and expected results, decision making models help demystify the process of making choices. This is particularly relevant in today's fast-paced world where quick, yet effective, decision making is essential for success.
The Concept of Decision Making
The essence of decision making involves choosing a course of action from multiple alternatives. This process can be simple or complex, depending on the nature of the decision and the stakes involved. Fundamentally, decision making relies on identifying problems, generating options, evaluating those options, and ultimately selecting the one that best addresses the issue at hand.
Understanding this process is crucial. When we grasp how decisions are made, we can better analyze our choices and those of others. Decision making is not just an intellectual exercise; it is a skillful art that blends analysis, intuition, and judgment.
Key elements of decision making include:
- Identifying the decision to be made: Clearly defining the issue is the first step.
- Gathering information: This includes data about possible options and potential outcomes.
- Evaluating alternatives: Weighing the pros and cons of various choices helps clarify the best path forward.
- Making the choice: Selecting the option that aligns with values and goals.
- Implementing the decision: Turning the chosen option into action while ensuring resources are allocated effectively.
- Reviewing the decision: After implementation, evaluating outcomes fosters learning for future decisions.
A comprehensive view of decision making encompasses both rational and emotional elements. This reflects the human condition, recognizing that decisions are often influenced not only by logic but also by biases and emotions.
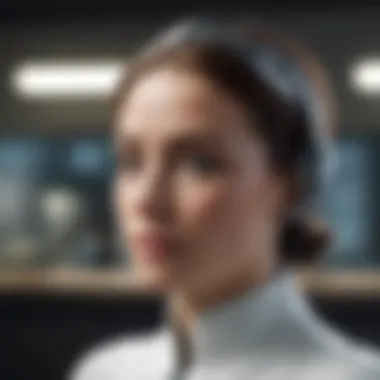
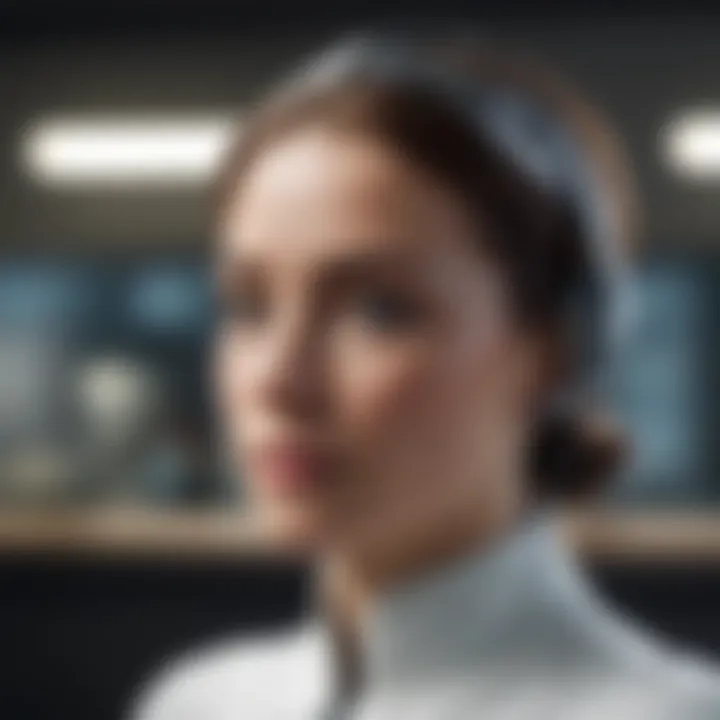
What Constitutes a Decision Making Model
A decision making model is a structured approach that outlines how decisions can be made systematically. It often consists of specific components like stages, processes, and methodologies designed to enhance understanding and effectiveness in decision making.
Common attributes of decision making models include:
- Frameworks: These are visual tools that represent the decision making process step-by-step.
- Assumptions: Each model operates based on certain premises about how decisions are made.
- Outcomes: These models help predict the likely results of different choices, which is critical in risk management.
- Flexibility: Effective models allow for variations depending on the context and specific circumstances surrounding the decision.
Models such as the Rational Decision Making Model, Bounded Rationality Model, and Intuitive Decision Making Model serve distinct purposes and applications.
In summary, defining decision making models is crucial for enhancing the decision-making process. It offers clarity, structure, and insight into choices, supporting better outcomes in both personal and professional contexts.
Historical Context of Decision Making Models
Understanding the historical context of decision making models enriches our grasp of their evolution and significance in contemporary settings. The frameworks and theories that govern decision processes today are deeply rooted in historical developments. An exploration of these models reveals not only the shifts in thinking over time but also the impact of societal changes on decision-making practices. It highlights how decision making has become more structured, data-driven, and versatile, responding to the complexities in various fields such as business, psychology, and economics.
Evolution Over the Decades
The evolution of decision making models can be traced through several key phases, reflecting the changing landscape of knowledge and technology. In the early 20th century, decision making was mostly intuitive and lacked formal frameworks.
As businesses became more complex, particularly post-World War II, the need for systematic approaches grew. The introduction of the Rational Decision Making Model marked a significant advance, emphasizing logical analysis and structured methodologies. This model advocated for clearly defined steps in the decision-making process, such as identifying problems, evaluating alternatives, and selecting the optimal solution.
By the 1970s, scholars like Herbert Simon introduced the concept of bounded rationality. This model acknowledged the limitations of human cognition and urged for a more realistic approach to decision making, considering the constraints of time and information processing capacity.
The 21st century has witnessed a technolocal revolution in decision making. The advent of big data, artificial intelligence, and machine learning has transformed how organizations and individuals approach decisions. These technological advancements enable real-time analysis of vast datasets, fostering data-driven outcomes that were previously unattainable.
Key Historical Theorists and Their Contributions
Significant theorists have shaped the landscape of decision making over time. Here are a few key figures and their notable contributions:
- Herbert Simon: He highlighted the concept of bounded rationality, fundamentally changing how decision making is viewed in the context of inheirent cognitive limitations.
- Daniel Kahneman and Amos Tversky: Their work on behavioral economics introduced insights about cognitive biases and their effects on decision making, which led to the development of models that integrate psychological aspects.
- John von Neumann and Oskar Morgenstern: They laid the groundwork for game theory, providing a strategic lens through which to analyze competitive decision-making scenarios.
These contributors, among others, have enriched our understanding of decision making by emphasizing the relevance of human psychology and social dynamics. Their work underscores the importance of adapting decision-making strategies to accommodate evolving contexts, ensuring better outcomes in both organizational and individual decision-making scenarios.
"The history of decision making is not just about models, but also about understanding the human elements that influence choices."
In summary, the historical context of decision making models is not merely an academic endeavor. It serves as a vital reference point for evaluating modern practices and prepares professionals to engage with future developments effectively.
Types of Decision Making Models
Understanding the variety of decision making models is essential for effective strategic planning, both in organizations and personal scenarios. Each model provides a framework that addresses the complexity and nuance inherent in decision processes. By learning about the different models, professionals can select the most appropriate approach for particular situations. This equips them with the necessary tools to enhance both individual and collective decisions.
Rational Decision Making Model
The rational decision making model is one of the most fundamental approaches in decision theory. This model is predicated on the idea that individuals make decisions by evaluating all available information systematically. The goal is to arrive at the optimal choice by weighing all possible alternatives against a set of criteria. The steps of this model typically include:
- Identifying the problem
- Gathering relevant information
- Evaluating alternatives
- Making a decision
- Implementing the decision
- Monitoring outcomes
One important advantage of this model is its structure, which encourages thorough analysis. However, it can be time-consuming and assumes that decision makers are fully rational, which is not always the case in real-world contexts.
Bounded Rationality Model
Herbert Simon introduced the bounded rationality model as a critique of the rational decision making model. This approach acknowledges the limitations in human cognitive abilities and the availability of information. The bounded rationality model suggests that individuals often rely on heuristics—mental shortcuts—to simplify decision making.
This model highlights several important points:
- Decisions are often made under constraints.
- There is an inherent complexity in assessing all available information.
- Decision makers may settle for a satisfactory option rather than the optimal one.
While this model reflects a more realistic view of decision making, it also points to the need for awareness of biases that can influence choices.
Intuitive Decision Making Model
Intuitive decision making relies on instinct and gut feelings rather than structured analytical processes. Intuition can be informed by experience, emotions, and rapid information processing. This model can be particularly valuable in fast-paced environments where time constraints limit deliberation.
Some characteristics of this model include:
- Quick decision-making capability
- Potentially less resource-intensive
- Reliance on prior knowledge and experience
However, while intuition can lead to swift choices, it is also susceptible to error and biases, making it crucial that professionals retain a level of skepticism regarding their gut feelings.
Creative Decision Making Model
The creative decision making model emphasizes innovation and out-of-the-box thinking. This model encourages brainstorming and the exploration of novel solutions. Teams or individuals employing this method often engage in either divergent or convergent thinking to generate a range of ideas or to narrow down options effectively.
Key elements include:
- Emphasis on originality
- Encouragement of risk-taking
- Flexibility in thought processes
Though creativity can foster unique solutions, it is vital to balance this with practical considerations and feasibility to ensure decisions are implementable.
Participative Decision Making Model
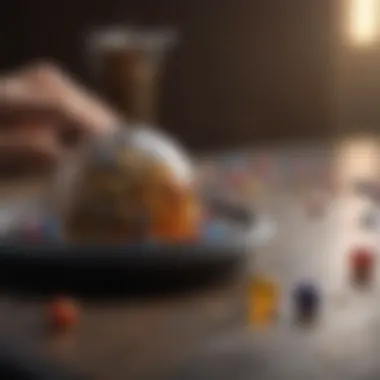
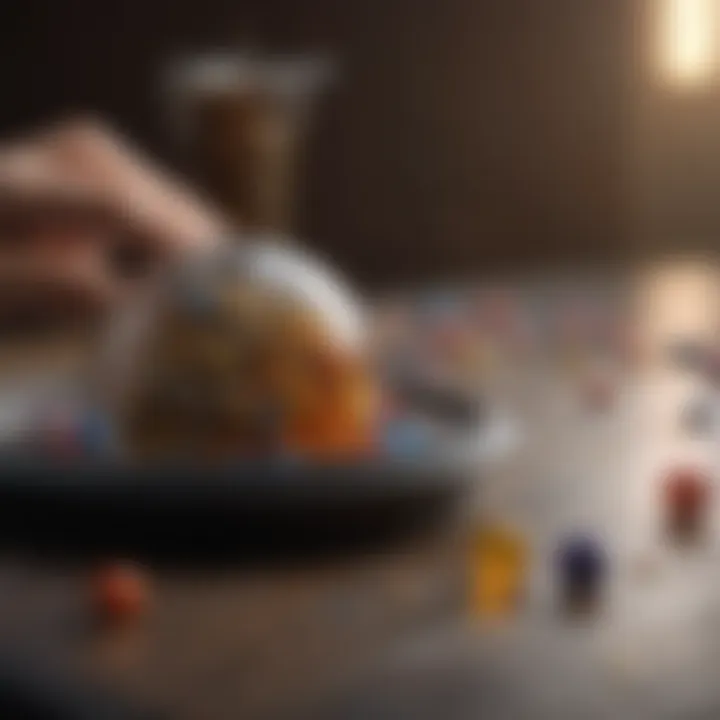
The participative decision making model involves collaboration among team members in the decision-making process. This inclusiveness promotes collective intelligence and taps into diverse perspectives. Engaging multiple stakeholders can enhance buy-in and ensure that varying insights are considered.
Some advantages include:
- Improved morale and motivation among participants
- Better quality of decisions through diverse inputs
- Enhanced communication and trust within teams
Nonetheless, it can sometimes slow down decision making, as reaching consensus and accommodating differing views requires time and effort.
In summary, these models can significantly influence how choices are made in various scenarios. Understanding their unique features enables professionals to adopt the most suitable approach based on context, problem complexity, and available resources.
The Role of Data in Decision Making
Data is an increasingly pivotal element in the contemporary landscape of decision making. Its role cannot be overstated, as effective decision making hinges on the accurate interpretation and application of data. Companies and individuals alike leverage data to substantiate their choices, making their decisions not just informed, but strategically sound. The integration of data into decision-making processes allows for a clearer view of the scenarios at hand, enhancing both intuition and rationality in choices.
Importance of Data in Modern Models
In modern decision making models, data serves as the backbone. This holds true across various contexts, whether in business, healthcare, education, or personal life. The reliance on data has transformed how decisions are made. Several points underscore the importance of data:
- Accuracy and Reliability: Decisions backed by factual data are usually more reliable. These decisions can be evaluated, tested, and, if necessary, corrected based on what the data indicates.
- Trends Identification: Data analysis helps identify trends that can influence future decisions. For instance, observing purchasing trends in retail can significantly inform inventory decisions.
- Predictive Analysis: Data enables predictive modeling, which is crucial for anticipating outcomes based on historical data. This is particularly important in sectors like finance, where market trends shape investment strategies.
- Efficiency Improvement: Data-driven decisions often result in improved operational efficiency. When organizations utilize data to streamline their processes, they enhance productivity and reduce waste.
- Enhanced Customer Understanding: In a consumer-driven market, understanding customers through data helps tailor products and services to better meet their needs.
In sum, data enhances decision-making models by providing clarity, insight, and direction that is often unavailable without thorough analysis.
Data Analysis Techniques in Decision Making
Understanding how to analyze data effectively is a crucial skill in decision making. Several techniques are commonly employed:
- Descriptive Analysis: This technique summarizes past data to provide insights into what has occurred. It is especially useful for organizational performance reviews.
- Diagnostic Analysis: Here, analysts investigate data to understand why something happened. This can involve root cause analysis, which is valuable in problem-solving.
- Predictive Analysis: Using statistical models and machine learning, this type projects future outcomes based on historical data. It allows decision-makers to anticipate challenges or opportunities.
- Prescriptive Analysis: This technique goes a step further by recommending actions based on predictive analysis. It helps in optimizing the decision-making process.
The combination of these techniques facilitates an informed decision-making process that is not only responsive to current conditions but also proactive in predicting and preparing for future scenarios. By using data effectively, individuals and organizations can navigate complexities efficiently.
Behavioral Science and Decision Making
Behavioral science plays a pivotal role in understanding decision-making processes. It examines how psychological, social, and emotional factors influence the way individuals and groups make choices. This intersection of psychology, sociology, and economics provides a framework to comprehend the often irrational behaviors observed in decision-making scenarios.
In this context, the significance of behavioral science cannot be overstated. It addresses behavioral biases, which can skew decisions and lead to suboptimal outcomes. Moving beyond traditional models_ which often assume rationality_, behavioral insights shed light on the real-world complexities of human choices.
The application of behavioral science in decision-making models offers several benefits. It enhances the precision of predictions regarding how people will behave in different situations. By incorporating these insights, organizations can develop more effective strategies that align with actual human behavior rather than idealized models.
Considerations surrounding behavioral science include the challenge of implementing these insights in practical settings. There is a need for extensive training and development to ensure that decision-makers can apply these findings effectively.
Behavioral Biases in Decision Making
Behavioral biases profoundly impact decision-making. Some common biases include confirmation bias, anchoring, and loss aversion.
- Confirmation bias often leads individuals to search for, interpret, and remember information in a way that confirms their preexisting beliefs. This can significantly hinder objective decision-making.
- Anchoring occurs when individuals rely too heavily on the first piece of information they encounter. For example, the initial price of a product can set an anchor that influences future valuations.
- Loss aversion refers to the tendency to prefer avoiding losses rather than acquiring equivalent gains. In decision-making, this bias can cause individuals to hold onto losing investments or avoid necessary risks.
Understanding these biases can help organizations identify potential weaknesses in their decision-making processes. Addressing these biases requires awareness and training to create environments where better decision-making can thrive.
Incorporating Behavioral Insights into Decision Models
Incorporating behavioral insights into decision models involves recognizing the limitations of traditional approaches. This means adapting models to account for human behavior and its inherent complexities. It requires a shift from purely rational models to those that integrate psychological factors.
One effective method is through the design of nudges. Nudges are subtle changes in the way choices are presented that can significantly influence outcomes without restricting freedom of choice. For instance, changing the default option in a retirement savings plan to an opt-out rather than opt-in can lead to higher participation rates.
Organizations should consider using
- Behavioral data to identify trends and patterns that predict decision-making.
- Training programs that emphasize awareness of biases among employees.
- Simulations and role-playing scenarios to illustrate the effects of biases in real-world contexts.
By integrating these insights, organizations create decision models that not only reflect how people should make decisions but, more importantly, how they actually do.
"Understanding the nuances of human behavior leads to more effective decision-making strategies."
Ultimately, enriching decision-making frameworks with behavioral science promotes better organizational outcomes and personal decision-making efficacy.
Technological Integration in Decision Making Models
In today’s fast-paced environment, the integration of technology into decision-making models is no longer optional. It has become a vital component for leaders and organizations aiming for efficiency and strategic advantage. Enhanced data processing capabilities, more accurate predictions, and better analytical frameworks have transformed how decisions are made. The synergy of human intellect and technological prowess creates a more robust decision-making landscape.
Impact of AI on Decision Making
Artificial Intelligence (AI) has reshaped the decision-making models significantly. By leveraging large datasets, AI can identify patterns that may not be immediately obvious to human analysts. This ability allows for faster decisions based on data rather than intuition alone. AI tools can assist in predictive analytics, risk assessment, and identifying opportunities that could have otherwise gone unnoticed.
Moreover, AI helps in automating routine decisions, enabling human resources to focus on more complex issues. It can also simulate various scenarios and outcomes, providing a clearer understanding of potential impacts before taking action.
"The infusion of AI into decision-making offers unprecedented insights, transforming decision-making nuances into data-driven practices that enhance accuracy and reliability."
Using Machine Learning for Enhanced Decision Making
Machine Learning (ML), a subset of AI, introduces advanced algorithms that improve over time. It enables systems to learn from previous data without explicit programming. This adaptive learning is paramount for enhancing decision-making processes. By applying ML models, organizations can forecast shifts in market dynamics, customer behaviors, and optimize operational efficiency.
In practical scenarios, ML algorithms can analyze past sales data to predict future trends. They can also enhance customer segmentation, enabling more targeted marketing strategies. The continuous feedback loop in ML ensures that decisions can be refined based on real-time performance metrics.
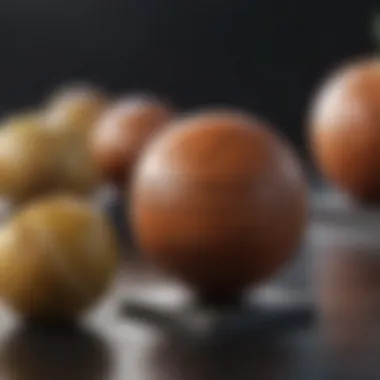
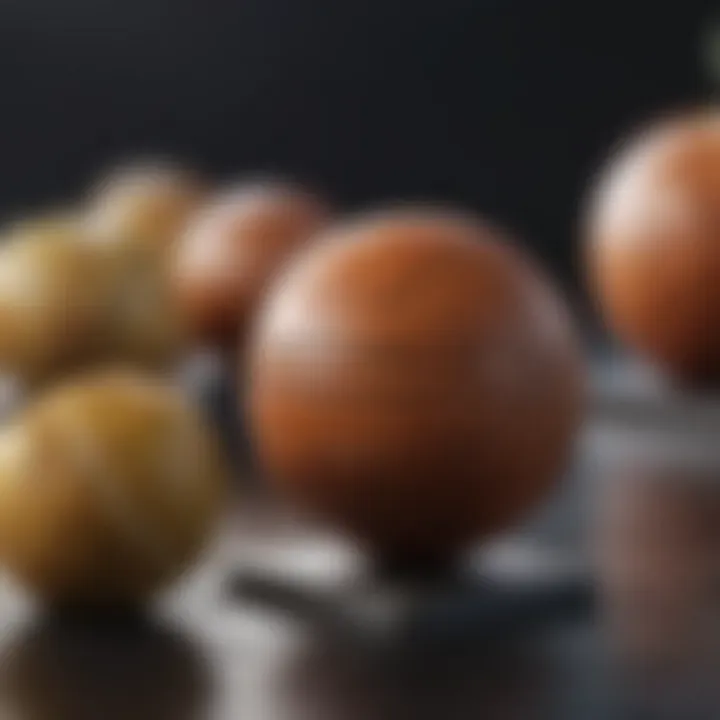
Additionally, organizations must consider some challenges. Data quality and ethics in AI use are critical topics that require attention. An organization can only make informed choices if the underlying data is reliable and unbiased. Hence, maintaining integrity in data sources is key to successful technological integration in decision making.
In summary, technological integration, particularly through AI and ML, represents a crucial evolution in the effectiveness of decision-making models. It not only enhances the ability to make informed choices but also prepares organizations to navigate increasingly complex landscapes with confidence.
Practical Applications of Decision Making Models
Understanding practical applications of decision making models is crucial for both individuals and organizations. These models provide a structured approach to making choices, which enhances effectiveness in various contexts. From strategic planning to everyday decision-making scenarios, these models serve as vital tools that can significantly improve outcomes and promote efficiency. Using the right model in the right situation can often lead to better results by reducing uncertainty and clarifying objectives.
Case Studies in Organizational Decision Making
Case studies illustrate the effectiveness of decision making models in real-world scenarios. Organizations often face complex challenges that require thoughtful analysis and strategic responses. For example, consider how Google employs decision making models in product development. Their use of the rational decision-making model enables teams to systematically analyze options for new features or services before committing resources. This structured approach helps them prioritize initiatives based on data-driven insights and customer needs.
In another instance, Procter & Gamble utilizes a participative decision-making model. By involving diverse teams in their decision processes, they harness collective knowledge and creativity. This not only enhances team morale but also leads to more innovative solutions, as team members feel more invested in the outcomes. Case studies like these highlight the tangible benefits decision making models can offer, providing clear examples of improved efficiency and effectiveness in organizational contexts.
Individual Decision Making Scenarios
On an individual level, decision making models play a significant role in enhancing personal effectiveness. Take, for example, the intuitive decision-making model. Individuals often rely on their gut feelings when faced with choices in daily life. This model can be particularly useful in situations where time is limited, or information is scarce. However, it is essential to balance intuition with analytical data when making critical life choices.
In contrast, individuals may also benefit from applying the rational decision-making model when considering long-term career options. By laying out potential paths, evaluating possible outcomes, and assessing risks, a person can make a more informed choice. In both personal and professional contexts, understanding which model to apply allows individuals to navigate their decisions with clarity and confidence.
"The application of decision making models can empower individuals and organizations alike, enabling them to approach challenges with structured thought and informed choices."
In summary, practical applications of decision making models showcase their importance in navigating both organizational and individual decisions. Since these models offer frameworks that facilitate clearer thinking and better outcomes, mastering them can be beneficial for anyone looking to enhance their decision-making capacity.
Challenges in Decision Making Models
The realm of decision making is fraught with challenges that can impede effectiveness. Addressing these challenges is crucial as they impact both individuals and organizations. In this section, we focus on two main hurdles: the complexity of real-world decisions and the limitations of traditional models. Each of these elements highlights the multifaceted nature of decision-making processes and their implications for practical applications.
Complexity of Real-World Decisions
Real-world decisions are rarely straightforward. Factors such as unpredictability, incomplete information, and emotional biases complicate the decision-making process. Decisions in a business context may involve numerous stakeholders with divergent interests. The intertwining of various elements makes it challenging to anticipate outcomes and assess options effectively.
In many cases, managers confront dilemmas where quantitative data conflict with qualitative insights. For instance, a decision based purely on financial metrics may not consider employee morale, customer satisfaction, or brand reputation. This divergence creates a tension that requires careful navigation.
"Real-world decisions often involve ambiguity and uncertain outcomes, pushing decision makers to rely on their judgment."
Furthermore, external factors such as market fluctuations or regulatory changes can further muddy the waters. Input from various departments must be synthesized, taking into account the perspectives of finance, marketing, operations, and human resources. This often leads to decision fatigue and delays, which can hinder progress.
Limitations of Traditional Models
Traditional decision-making models, while valuable, often fall short in addressing the complexities of contemporary challenges. Models like the Rational Decision Making Model assume a level of certainty and linearity that does not hold in many real scenarios. Under the assumption that decision makers can identify all possible options and outcomes, these traditional models can lead to oversimplification.
Key limitations include:
- Assumptions of Rationality: Many models presume that individuals make decisions in a rational fashion. However, behavioral science has shown that emotional and cognitive biases play a significant role in decision-making.
- Inflexibility: Traditional models often do not adapt well to changing circumstances, which is a frequent occurrence in dynamic business environments.
- Overreliance on Data: While data is essential, traditional models may emphasize quantitative analysis to the detriment of qualitative insights. This can result in a narrow view that does not account for human factors.
Future Trends in Decision Making Models
The evolution of decision-making models is closely linked with advancements in technology and shifting paradigms in how organizations function. As the complexity of decisions increases, so does the need for more sophisticated models. Understanding future trends in decision making is crucial for both personal and professional growth. It allows individuals and organizations to prepare for changes that could overhaul their current methodologies. The emerging paradigms are more focused on adaptability and real-time analysis, reshaping the decision-making landscape significantly.
Emerging Technologies Shaping Decision Making
Emerging technologies play a pivotal role in the evolution of decision-making processes. Tools like artificial intelligence, big data analytics, and blockchain are not just buzzwords; they are driving forces in transforming how we make decisions. Here are some key aspects to consider:
- Artificial Intelligence: AI systems can analyze vast amounts of data far quicker than human capability. This speed allows organizations to make informed decisions swiftly, reducing potential delays in critical areas.
- Big Data: The ability to harness big data enables a more nuanced understanding of trends and patterns. Organizations that utilize data analytics can not only predict outcomes but anticipate them, leading to more proactive decision-making.
- Blockchain: Transparency and security offered by blockchain technology can bolster trust in decision-making processes, particularly in areas that require accountability.
The integration of these technologies is reshaping decision-making models, creating a more informed and responsive approach. As organizations continue to adopt these tools, the models will focus on real-time updates and data integrity.
The Shift Towards Dynamic Decision Making Models
The concept of dynamic decision-making models is emerging as organizations recognize the limitations of static approaches. Traditional models often provide a one-size-fits-all solution that may not suit fast-paced environments.
Dynamic models respond to changes in real time and accommodate new data, making them particularly beneficial in volatile industries. Some characteristics include:
- Adaptability: This allows organizations to pivot quickly when new information arises, ensuring decisions are based on the latest data.
- Continuous Improvement: Learning from past decisions becomes an inherent part of the process, fostering a culture of growth and adaptation.
- Inclusivity: Engaging various stakeholders in the decision-making process can lead to better insights and outcomes.
As organizations face uncertainty, the flexibility inherent in dynamic models will likely become a necessity, not just a choice. This shift represents a profound change in thinking, focusing on continuous improvement and stakeholder engagement.
"The future of decision making models lies in their capacity to adapt and integrate emerging technologies for real-time insights and actions."
Through understanding these trends, professionals can equip themselves with the knowledge needed to navigate an increasingly complex world. Failure to adapt to these developing models may mean falling behind in an environment that is rapidly evolving.
Culmination
The conclusion of this comprehensive guide on decision-making models is essential in tying together the various elements covered throughout the article. It serves as both a summary and a reflection on the critical relevance of understanding these models in diverse contexts. Decision-making, a fundamental aspect of human behavior, is influenced by numerous factors including data, technology, and human psychology. Therefore, grasping the nuances of different models aids individuals and organizations in navigating complex environments more effectively.
Summary of Key Insights
A conclusive overview unveils several key insights:
- Diverse Models Exist: There are various decision-making models, each with unique strengths and weaknesses. The Rational and Bounded Rationality models provide frameworks for logical analysis, while the Intuitive and Creative models highlight the importance of instinct and imagination in the decision process.
- Importance of Data: In modern settings, data plays a pivotal role. The integration of data analysis techniques into decision-making allows individuals and organizations to make informed choices backed by evidence.
- Behavioral Aspects Matter: Understanding behavioral biases is crucial. Incorporating insights from behavioral science into decision models can improve outcomes by accounting for irrationalities that often hinder effective decision-making.
- Technological Integration is Crucial: The rise of AI and machine learning has transformed decision-making practices. These technologies refine decision quality and speed, which is vital in fast-paced environments.
- Future is Dynamic: Decision-making models are evolving. The shift towards dynamic models reflects the need for adaptability in an ever-changing world. Organizations need to embrace this momentum for sustainable decision processes.
Implications for Personal and Organizational Growth
The implications of these insights are profound. For individuals, understanding decision-making models can lead to improved personal effectiveness. By recognizing which model to apply in various situations, one can enhance their problem-solving capabilities. Decision-making becomes more systematic, reducing the occurrence of errors driven by cognitive biases.
For organizations, the strategic application of decision-making models leads to better planning and implementation processes. Decision transparency fosters collaboration, ultimately improving team performance. Organizations equipped with advanced models can pivot more quickly in response to market changes or internal challenges. It becomes easier to harness collective intelligence, improving overall outcomes.